NVIDIA GTC 2025 主题演讲
文章语言:
简
繁
EN
Share
Minutes
原文
会议摘要
The dialogue highlights NVIDIA's significant strides in AI technology, emphasizing the efficiency and performance of architectures like Hopper and Blackwell, the importance of energy efficiency in data centers, and innovations such as 4-bit floating-point precision and silicon photonics to address networking challenges. It also introduces upcoming architectures, AI factories, and new product lines designed for data scientists and researchers.
会议速览

Tokens play a crucial role in detecting diseases early, decoding the intricacies of life, and understanding biological functions, aiding in the conservation of valued species.

At GTC, the discussion highlights the transformative impact of AI and robotics in maximizing potential and enhancing human capabilities, marking the beginning of a new era in technology exploration.

Nvidia highlights significant advancements in artificial intelligence, showcasing how AI has transformed computer graphics and computing models, leading to generative computing and enhanced performance in various industries.

The recent breakthroughs in agentic AI, characterized by its ability to perceive, reason, and act, and physical AI, which understands the physical world, are transforming computing layers. These advancements open new market opportunities and enhance problem-solving capabilities across various industries.

The dialogue discusses the three fundamental challenges in AI development: solving the data problem, training models without human intervention, and achieving scalable algorithms. It highlights the significant increase in computational requirements due to agentic AI's ability to reason, breaking problems down step-by-step, which generates a substantially higher number of tokens compared to previous AI models.

The dialogue highlights the significant increase in computational requirements for AI models, emphasizing the role of reinforcement learning and synthetic data generation in teaching AI to reason. It discusses the challenge of generating vast amounts of data for training, particularly without relying heavily on human input, and showcases the potential of reinforcement learning in solving complex problems across various domains. The speaker underscores the industry's response to these challenges, indicating a major shift in AI training methodologies.

The dialogue highlights the significant growth in AI infrastructure, particularly among the top four CSPs (Amazon, Azure, GCP, Oci), noting the increased demand and computational needs for AI models. It predicts a trillion-dollar data center build-out by the end of the decade, driven by the shift from general-purpose computing to AI-accelerated computing and the recognition of capital investment in software's future. This transformation is characterized by the emergence of AI factories focused on generative computing for various applications.

The dialogue highlights NVIDIA's development and impact of various accelerated libraries, including cuNumeric, cuLitho, and cuOpt, which significantly enhance performance in fields such as computational lithography, 5G radio processing, and mathematical optimization, aiming to open-source some of these tools to further accelerate industry advancements.

The announcement highlights significant advancements in accelerated computing through partnerships with major systems companies, introducing QDSS sparse solvers and accelerated libraries for structured data, physics simulations, and more, marking a tipping point in the industry's shift towards CUDA-powered solutions.

The speaker discusses the evolution of AI, emphasizing its origins in the cloud due to infrastructure and computational needs. They highlight the importance of full-stack technology and the role of cloud service providers in nurturing AI development. The speaker expresses excitement about AI's expansion into various sectors, including enterprise, manufacturing, robotics, and self-driving cars, and mentions the emergence of GPU clouds. They particularly focus on the transformative potential of AI in radio networks and edge computing, announcing a collaboration with major companies to build a full stack for radio networks in the U.S., aiming to revolutionize communications and adapt radio signals using AI and reinforcement learning.

NVIDIA has been deeply involved in the development of self-driving car technology for over a decade, partnering with major companies like Tesla and GM. Their technology, including GPUs and AI-driven systems, is utilized in both data centers and vehicles for training, simulation, and on-road operations. NVIDIA is particularly proud of its commitment to automotive safety, having developed a comprehensive safety assessment process for every line of code and securing over 1000 patents related to safety in autonomous vehicles. They employ advanced AI techniques such as model distillation, closed loop training, and synthetic data generation to enhance the adaptability and safety of autonomous vehicles, ensuring they can navigate complex scenarios robustly.

Nvidia achieves unprecedented scale-up in computer architecture through the Grace Blackwell MvLink 72 rack, marking a fundamental shift from integrated to disaggregated MvLink and from air-cooled to liquid-cooled systems, enabling a 1x flops supercomputer in a single rack.

The dialogue discusses the extreme computing challenge of AI inference, emphasizing the need for high efficiency and performance in generating tokens for AI responses. The speaker explains the significance of token generation speed and volume, highlighting the balance required between latency and throughput in AI systems to ensure optimal service quality, revenue, and profitability.

The discussion centers on the challenge of optimizing AI services to provide high-quality customer experience while maximizing data center efficiency and revenue. It explores the trade-offs between speed, computation power, bandwidth, and the complexity of AI models, highlighting a demo that compares traditional and reasoning models in solving complex problems.

The dialogue discusses optimizing AI models with trillions of parameters by distributing workloads across GPUs using tensor, pipeline, and expert parallelism. It highlights the importance of homogeneous architectures like NVLink for handling complex computing phases, such as context processing and decoding, in large language models.

NVIDIA introduces Dynamo, an open-source operating system designed to manage complex AI workloads in data centers, akin to orchestrating an AI factory, offering dynamic resource allocation for tasks like prefill and decoding.

The discussion explores the optimization of AI factory throughput and energy efficiency using the Hopper and Blackwell systems. It highlights the trade-offs between AI intelligence and volume production, emphasizing the importance of energy-efficient compute architectures in power-limited data centers. Innovations like MvLink 8 and Dynamo are introduced to enhance performance and efficiency.

The discussion highlights the significant performance improvements of Blackwell over Hopper in AI operations, emphasizing the balance between throughput and quality. Blackwell achieves 25 times the performance in one generation under ISO power conditions, demonstrating its superiority in handling next-generation workloads, particularly in reasoning models where it outperforms Hopper by 40 times.

The discussion highlights the superiority of investing in advanced technology, specifically comparing a 100 MW factory's efficiency using Hopper versus Blackwell chips, emphasizing significant improvements in production capacity and cost-effectiveness.

The dialogue highlights the complexity and scale of building state-of-the-art AI factories, emphasizing the use of digital twins for planning and optimization before physical construction. Nvidia's Omniverse Blueprint enables collaborative engineering teams to simulate and iterate designs efficiently, significantly reducing errors and accelerating the build-up process. The speaker also outlines a roadmap for upcoming AI infrastructure, including the Blackwell Ultra and Vera Rubin systems, showcasing the strategic planning required for such large-scale projects.

The dialogue discusses NVIDIA's GPU advancements, clarifying that each Blackwell chip comprises two GPU dies, and outlines future plans including the Ruben Ultra project, which aims for extreme scale-up with 576 MvLink connections and significant increases in performance and bandwidth. It also highlights the transition to Spectrum X networking technology, aiming to enhance Ethernet networks with Infiniband-like properties for better performance and manageability, exemplified by the creation of the largest single GPU cluster with Spectrum X.

The discussion outlines the challenges and solutions involved in scaling GPU usage in data centers, particularly focusing on the energy and cost implications of using transceivers. It introduces the invention of the world's first MRI micro mirror and the implementation of silicon photonics, which significantly reduce power consumption and enable the handling of massive GPU volumes. These advancements pave the way for more efficient, high-capacity data center architectures, aiming to save tens of megawatts of power and facilitating the deployment of millions of GPUs.

The transformation of enterprise computing through AI involves a new computing stack, including changes in processors, operating systems, and data access methods. AI agents will become integral to the digital workforce, significantly altering how enterprises operate and necessitating the development of a new line of computers.

A new line of high-performance computers, including the DGX Station and Spark, is announced for data scientists and researchers, marking the era of AI computing. These systems, capable of 20 petaflops and equipped with 72 CPU cores, HBM memory, and PCI Express slots, will be manufactured by major OEMs like HP, Dell, Lenovo, and Asus. The company is also revolutionizing computing, networking, and storage, introducing an open-source AI model that can be run on various platforms, including the new computers and in the cloud. Additionally, partnerships with global companies are highlighted for integrating AI frameworks. The discussion shifts to robotics, emphasizing the need for autonomous robots due to a global labor shortage. Nvidia's technology enables a continuous loop of robot AI simulation, training, testing, and real-world experience, with the introduction of Isaac GRO N1, a generalist foundation model for humanoid robots, showcasing advancements in embodied AI and robotics.

The core challenges in AI include data creation, model architecture, and scaling laws. To address these in robotics, Omniverse serves as the operating system for physical AI, enhanced by Cosmos for generating infinite, grounded environments. Newton, a collaboration between DeepMind, Disney Research, and Nvidia, introduces a specialized physics engine designed for fine-grained simulations, accelerating AI training for robotics.

After showcasing advanced robotics and AI technologies, including real-time simulation and robot training methods, the speaker announces that Group N 1 robotics platform is now open sourced, highlighting significant progress in AI and robotics. The event also covers the production status of Blackwell, emphasizing the increasing demand for AI computation and infrastructure for cloud, enterprise, and robots.
要点回答
Q:What are the capabilities of AI as emphasized in the speech?
A:AI can now help in detecting diseases before they take hold, understanding the language of life, protecting noble creatures, turning potential into plenty, harvesting bounty, teaching robots to move with joy, lending a helping hand, and putting life within reach.
Q:What is the importance of GTC and how does it relate to Nvidia?
A:GTC is an event associated with Nvidia, and it signifies a gathering where various industries come together to discuss the impact of AI. GTC is also a platform where Nvidia highlights its developments, such as AI and its role in computer graphics and computing.
Q:¿Cómo ha impactado la inteligencia artificial en los productos de Nvidia como GForce y GPUs?
How has artificial intelligence impacted Nvidia's products like GForce and GPUs?
A:AI has led to significant advancements in Nvidia's products, such as GForce, which experienced a revolution in computer graphics through AI integration. The new generation of GPUs, like the 50 90, showcases improvements in volume, energy dissipation, and performance thanks to AI.
Q:What are the two types of AI mentioned and what sets them apart?
A:The two types of AI mentioned are generative AI and agentic AI. Generative AI is about teaching AI to translate between different modalities like text to image, while agentic AI refers to AI that has agency, can perceive context, reason, and plan actions, making it useful for tasks requiring multimodality information and problem-solving.
Q:The role of physical AI in robotics is to enable machines to perceive and interact with their environment through physical and sensory manipulation.
A:Physical AI enables robotics by providing AI systems with understanding of the physical world, including concepts like friction, inertia, cause and effect, and object permanence. This understanding aids robots in performing tasks that require physical interaction with their environment.
Q:How has the computational demand for AI changed with the introduction of agentic AI?
A:With the advent of agentic AI, which is fundamentally about reasoning, the computational requirement for AI has increased substantially. AI that can reason step-by-step, such as AI using a chain of thought and best of n assistancy checking, generates a sequence of tokens that represent steps in the reasoning process, resulting in a much higher computational requirement compared to traditional AI models.
Q:Cuáles son los tres desafíos fundamentales en IA según el discurso?
What are the three fundamental challenges in AI according to the speech?
A:The three fundamental challenges in AI are solving the data problem (AI needs data to learn), solving the training problem without human in the loop (AI should be able to learn at super-human rates and at a scale humans cannot match), and scaling (AI needs to become smarter as more resources are provided, following a scaling law).
Q:What is the importance of the Hopper and Blackwell shipments that were mentioned?
A:The Hopper and Blackwell shipments signify the growth in AI infrastructure and the increasing computational power needed to support AI models, particularly those with reasoning capabilities. The data indicates a significant rise in capital expense for data centers and suggests that the demand for AI is driving substantial investment in computing infrastructure.
Q:¿Cuáles son las dos dinámicas que actualmente están ocurriendo en el mundo de la informática?
A:The two dynamics are: 1) A transition in computing from general-purpose computing to machine learning software running on accelerators and GPUs, evidenced by the shift in data center build-outs. 2) An increase in recognition that the future of software requires significant capital investment.
Q:What is meant by the computer becoming a token generator?
A:The speaker is referring to a shift from traditional computing where software is written and run on computers, to a new model where computers generate the necessary tokens for running software, transforming the role of computers from running files to generating data.
Q:Can you please provide more context for this sentence? It is quite vague.
A:The speaker's favorite slide is about the various libraries that are crucial for accelerated computing, which is the main focus of the GPU Technology Conference (GTC). It emphasizes the importance of these libraries in making AI and other applications possible.
Q:Por qué se necesitan bibliotecas especializadas como Cuda para campos fuera de la inteligencia artificial?
A:Specialized libraries like Cuda are needed for fields outside of AI because they optimize existing frameworks for different scientific fields such as physics, biology, and quantum physics, enabling the acceleration of processes that are essential in those industries.
Q:What impact will artificial intelligence have on communications and radio networks?
A:AI will significantly improve the efficiency and adaptability of radio networks by using techniques like reinforcement learning to optimize the use of radio signals in changing environments and traffic conditions.
Q:Cómo está revolucionando la inteligencia artificial el procesamiento de video y los gráficos en 3D?
How is artificial intelligence revolutionizing video processing and 3D graphics?
A:AI is enhancing video processing and 3D graphics by applying similar advancements that have been made in other areas, which likely include improvements in quality, efficiency, and contextual understanding.
Q:Which industry was the earliest to adopt AI, and how has it been integrated?
A:The earliest industry to adopt AI is autonomous vehicles. Nvidia has been instrumental in this development by providing technology that is used by many self-driving car companies, either in the data centers or directly in the vehicles themselves.
Q:¿Quién ha elegido Nvidia para su futuro flota de autos autónomos?
Who has chosen Nvidia for their future self-driving car fleet?
A:GM (General Motors) has chosen Nvidia to partner with them for building their future self-driving car fleet.
Q:What is the role of safety in GM's AI development?
A:Safety is a critical aspect of GM's AI development, with a focus on ensuring technology from silicon to systems is deeply ingrained in every part of the development process. GM has filed over 1,000 patents related to safety, including assessments of every line of code to ensure diversity, transparency, and explainability.
Q:Comment Nvidia soutient-il le développement de véhicules autonomes (AV) ?
How is Nvidia supporting the development of self-driving vehicles (AVs)?
A:Nvidia is supporting the development of AVs with its technologies such as Omniverse and Nvidia Autopilot, which includes capabilities like end-to-end trainable AI systems, model distillation, closed-loop training, and synthetic data generation. This helps in training AI systems, simulating driving scenarios, and generating driving knowledge that improves the robustness and adaptability of AVs.
Q:¿Qué innovaciones ha hecho Nvidia en los centros de datos y la arquitectura de inteligencia artificial?
What innovations has Nvidia made in data centers and AI architecture?
A:Nvidia has made fundamental transitions in computer architecture with innovations like Grace Hopper, which is an AI supercomputer. It features an advanced system architecture with up to 8 GPUs in one Blackwell package, enhanced with features like Mv link 8, dual CPUs, and Infiniband. These innovations have allowed for scaling up before scaling out, creating more efficient data centers and enabling advanced AI capabilities.
Q:Cómo planea Nvidia abordar los desafíos de generar tokens para sistemas de inteligencia artificial?
How does Nvidia plan to address the challenges of generating tokens for AI systems?
A:Nvidia aims to address the challenges of generating tokens for AI systems by creating a highly efficient 'factory' that maximizes the product of tokens per second and response time. This involves balancing the computational flops and bandwidth with the latency requirements to optimize the AI system's performance and responsiveness.
Q:Traditional language models (LLMs) have limitations in solving complex problems due to their inability to understand context, lack of knowledge of specific domains, and inability to handle ambiguity.
A:Traditional LLMs are limited in their ability to solve complex problems as they may make mistakes even with foundational knowledge, like seating guests at a wedding table according to specific constraints.
Q:A reasoning model approaches complex problems differently from traditional Large Language Models (LLMs) by using logical and systematic reasoning processes to break down and analyze the problem, rather than relying solely on language processing and pattern recognition.
A:A reasoning model approaches complex problems by engaging in reasoning, trying multiple scenarios, and assessing its own answers, unlike traditional LLMs which may provide a one-shot response without the reasoning process.
Q:Why is problem-solving important in terms of computational resources?
A:Reasoning about problems is significant because it requires substantially more computational resources, as seen with the 8000+ tokens needed for a complex problem, compared to the simpler problem solved by a traditional LLM.
Q:Large language models pose challenges in terms of computational resources in terms of memory requirements, processing power, and energy consumption.
A:Large language models pose challenges due to their massive number of parameters, often in the trillions, which requires significant computational resources like terabytes per second of bandwidth and immense energy consumption.
Q:How does Nvidia's approach handle the computational requirements of these large models?
A:Nvidia's approach manages the computational demands by distributing the workload across GPUs using techniques like tensor parallelism, pipeline parallelism, and expert parallelism, optimizing for either high throughput or low latency, and employing in-flight batching and other techniques for efficient processing.
Q:What is the importance of Nvidia DynamO software in managing AI factories?
A:The Nvidia DynamO software is significant as it acts as the operating system for AI factories, managing complex operations including workload distribution, data processing, and AI model performance, to optimize AI production and efficiency.
Q:Simulations provide insights about the efficiency of AI factories.
A:Simulations provide insights into the efficiency of AI factories by showing how different configurations and techniques, such as the use of Nvidia DynamO and the balance between prefill and decode processes, affect the throughput and overall performance of the AI system.
Q:The term 'Pareto frontier' refers to the optimal trade-off between energy consumption and performance in data centers.
A:The 'Pareto frontier' refers to the most optimal configuration for a data center, representing the frontier of efficient energy usage where further improvement is not possible without sacrificing performance or power consumption.
Q:What implications does the demonstrated configuration change have for computer setup?
A:The demonstrated configuration change across the spectrum implies that the setup of computers can vary significantly depending on workload requirements, with different parts of the data center being optimized for different types of workloads.
Q:¿Cómo se compara Blackwell con Hopper en términos de rendimiento?
How does Blackwell compare to Hopper in terms of performance?
A:Blackwell is shown to be 40 times the performance of Hopper, indicating a substantial improvement in computational capability.
Q:The advantage of investing in Blackwell over Hopper, according to the speaker, is that Blackwell has a more stable financial track record and higher potential for growth.
A:The advantage of investing in Blackwell over Hopper is that Blackwell provides much greater performance and efficiency, with the implication that it is a more valuable investment due to the fast pace of technological advancement and the intensity of the workloads.
Q:An AI factory is a facility where artificial intelligence models and algorithms are developed, trained, and deployed for various applications and use cases.
A:An AI factory is a large-scale data center designed specifically for AI workloads, which includes an intricate network of components and requires sophisticated planning and engineering. The term 'digital twin' refers to a virtual representation of this data center that is built before the physical construction to optimize design and integration.
Q:What is the roadmap for future Nvidia products?
A:The roadmap presented for future Nvidia products includes the current full production of Blackwell, the upcoming transition to Blackwell Ultra in the second half of the year, Vera Rubin as the next product release in the following year with twice the performance of Grace, and the subsequent Ruben Ultra with extreme scale-up capabilities.
Q:Como se compara el nuevo producto Vera Rubin con las ofertas actuales?
A:Vera Rubin is positioned as the next evolution in product offerings, with double the performance of Grace and more bandwidth, as well as a 50 W CPU. It also signifies an update in nomenclature relating to the number of GPUs it supports.
Q:What does the term 'Blackwell' refer to in the speech and why is it important?
A:The term 'Blackwell' refers to a series of advanced chips designed to support AI workloads, featuring a combination of multiple GPUs on a single chip, which was initially misnamed in the speech but is clarified later on.
Q:Which technology enables the connection between GPUs and switches in a data center?
A:The technology that enables the connection between GPUs and switches in a data center is called Moander, which utilizes transceivers and lasers to transmit data from the GPU to the switch and then to subsequent switches.
Q:What is the main challenge in increasing the scale of computing resources in artificial intelligence, and how is Nvidia tackling it?
A:The main challenge in scaling up computing resources in AI is the energy consumption required to power the additional hardware. Nvidia is addressing this challenge by developing innovative technologies like silicon photonics and the invention of the MRI micro mirror, which together with other advances, allow for more efficient use of energy and the deployment of AI systems at scale without incurring excessive energy costs.
Q:¿Cómo afecta la invención del microespejo de resonancia magnética (MRI) al consumo de energía en los centros de datos?
A:The invention of the MRI (Micro-Resonant Mirror) micro mirror, which uses a waveguide, a resonant ring, and modulates energy to shut off or pass on light, helps save energy in data centers. It allows for the reduction of energy consumption by limiting the amount of light (and thus power) that goes through, which translates into a reduction of 180 MW of transceivers' energy usage for a large number of GPUs.
Q:The significance of the silicon photonic switch and related technology lies in its ability to efficiently and quickly route data signals using light on a silicon chip. This technology has the potential to greatly increase the speed and efficiency of data transmission in electronic devices, leading to faster and more reliable communication networks. Silicon photonic switches have the potential to revolutionize the way data is processed and transmitted, making them a key technology in the development of future communication systems.
A:The silicon photonic switch is significant because it enables the integration of photonic and electronic ICs, along with micro lenses and a fiber array, using TSMC's 3D co-packaged technology. This technology allows for the creation of an energy-efficient, high-performance switch that can be deployed in data centers and supports the scaling up to multi-million GPUs. It contributes to energy savings and enables the development of AI and enterprise computing infrastructure.
Q:How will Artificial Intelligence change the future of enterprise computing and digital workers?
A:AI will fundamentally change the future of enterprise computing by reinventing the computing stack, including processors, operating systems, applications, and the way they are orchestrated and run. It will lead to the rise of digital workers, with AI agents becoming a part of the workforce, anticipated to grow from a billion to ten billion as AI assistive tools for software engineers. AI will also revolutionize the entire computing stack to support the new needs of enterprise computing.
Q:Qual é a nova linha de computadores da Nvidia para IA, e quem se beneficiará deles?
A:Nvidia's new line of computers for AI includes a range of personal computers and workstations, such as the DGX Station, which offers 20 petaflops of computing power, 72 CPU cores, and HBM memory. These computers benefit data scientists and researchers around the world, including those in small to large enterprises, by providing powerful tools to advance AI development and research.
Q:Cuál es el enfoque de Nvidia para abordar los desafíos en robótica y inteligencia artificial para sistemas físicos?
A:Nvidia's approach to addressing the challenges in robotics and AI for physical systems includes creating a system called Omniverse, which serves as an operating system for physical AI. It includes the use of generative models like Cosmos, which can create diverse and controlled environments for training robots. Additionally, Nvidia partnered with DeepMind, Disney Research, and themselves to create a physics engine named Newton, which simulates real-world physics for training robots with tactile feedback and fine motor skills at super-real-time speeds.
Q:What does the release of Group N 1 signify for the robotics and AI community?
A:The release of Group N 1 signifies an advancement in the field of humanoid robots and AI. It is a general-purpose AI foundation model for humanoid robots that benefits from synthetic data generation, learning, and simulation. It allows developers to train robots across multiple tasks and environments, which is a significant step towards the integration and autonomy of robots in various industries.
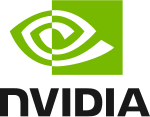
NVIDIA Corp.
Follow